The Essential Role of Image Annotation in Machine Learning
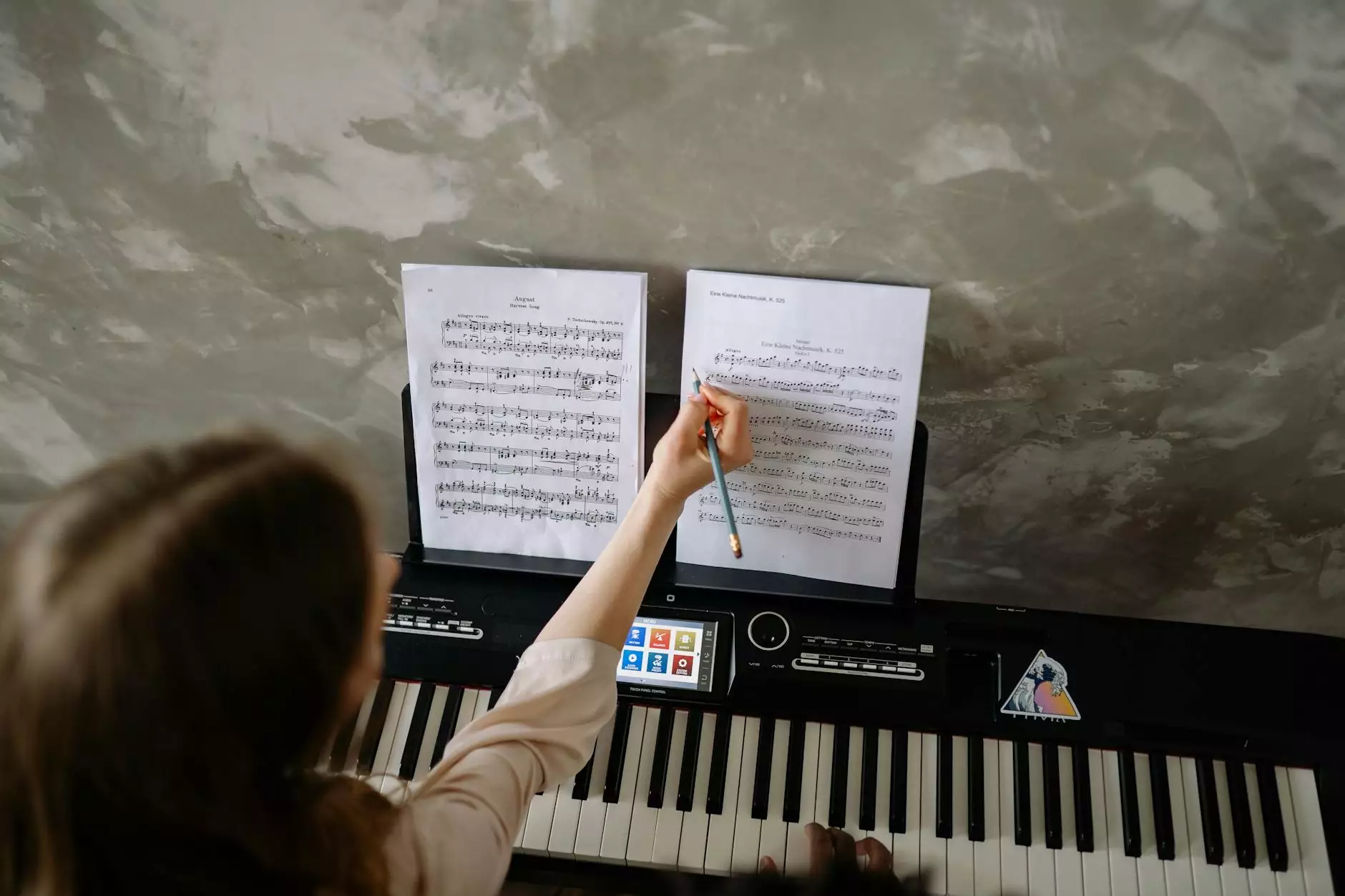
In an era where data reigns supreme, the ability to annotate images effectively has become vital for businesses leveraging machine learning. The process of image annotation serves as a cornerstone for training artificial intelligence algorithms, ensuring accuracy, reliability, and efficiency. In this comprehensive article, we will delve into the significance of image annotation in machine learning, explore various methods and tools, and highlight how Keylabs.ai stands at the forefront of this transformative technology.
Understanding Image Annotation
Image annotation is the procedure of identifying and labeling various elements within an image. This can range from marking the location of objects to categorizing images based on their contents. The application of image annotation is crucial, particularly in sectors such as autonomous driving, medical imaging, and e-commerce.
The Importance of Image Annotation in Machine Learning
The effectiveness of machine learning algorithms largely depends on the quality and quantity of the training data provided. Here are several key reasons why image annotation is indispensable in the realm of machine learning:
- Data Quality: High-quality annotations enhance the performance of machine learning models.
- Model Accuracy: Well-annotated images lead to more precise results, reducing error rates in predictions.
- Scalability: Large datasets require systematic annotation processes to maintain standardization.
- Enhanced User Experience: Businesses can provide better services and products through tailored machine learning models.
Types of Image Annotation Techniques
When it comes to image annotation for machine learning, there are several techniques that can be employed to label image data effectively. Below are some prevalent forms:
1. Bounding Box Annotation
Bounding box annotation involves drawing rectangles around objects in an image. This method is widely used in applications such as pedestrian detection, object recognition, and surveillance systems. Each box is labeled based on the object it encompasses, forming a dataset that is easily digestible by machine learning algorithms.
2. Polygon Annotation
While bounding boxes are effective, they often fail to capture the intricate shapes of objects. Polygon annotation addresses this by allowing for precise outlining of irregular shapes. This technique shines in scenarios like medical imaging, where exact contours are essential for diagnostic accuracy.
3. Semantic Segmentation
Semantic segmentation involves classifying each pixel in an image into a known category. This granular level of detail is essential for applications requiring high precision, such as autonomous driving and agricultural monitoring.
4. Instance Segmentation
A step further from semantic segmentation, instance segmentation not only classifies each pixel but also differentiates between various instances of the same object. This technique is particularly useful in complex environments, where multiple instances of the same object exist.
5. Keypoint Annotation
Keypoint annotation focuses on identifying and labeling specific points within images. For example, in facial recognition, keypoints might include the corners of the eyes, nose, and mouth. This method is fundamental in applications involving human pose detection and movement analysis.
How Keylabs.ai Enhances Image Annotation
At Keylabs.ai, we understand that the future of machine learning is rooted in the ability to process and analyze vast amounts of data accurately. Our data annotation platform is engineered to offer cutting-edge solutions that enhance the image annotation process. Here’s how we stand out:
Robust Annotation Tools
Keylabs.ai provides a suite of powerful tools designed to facilitate efficient and accurate image annotation. Our platform accommodates various annotation types, including bounding boxes, polygons, and keypoints, ensuring versatility for any project.
Collaboration and Scalability
The collaborative features of our platform allow teams to work seamlessly, providing oversight and management to maintain data quality. With the capacity to scale up annotation efforts, businesses can swiftly ramp up projects to meet growing demands.
Quality Assurance Mechanisms
We prioritize the integrity of our annotations. Our quality assurance systems employ both human and AI oversight to verify data accuracy, ensuring that businesses receive top-notch datasets for their machine learning endeavors.
Practical Applications of Image Annotation in Various Industries
The applications of image annotation are vast and diverse, spanning numerous industries. Here are some key sectors where image annotation in machine learning is making a significant impact:
1. Healthcare
In the healthcare sector, accurate image annotation is vital for medical imaging technologies. Annotated images facilitate the training of models that assist in diagnosing conditions from MRIs and CT scans, ultimately enhancing patient care.
2. Automotive
The automotive industry utilizes image annotation extensively in the development and training of autonomous vehicles. Annotated datasets help in teaching algorithms to recognize various traffic signs, pedestrians, and road conditions, contributing to safer driving experiences.
3. Retail and E-Commerce
Retailers use image annotation to categorize products effectively and enhance shopping experiences. By employing machine learning with well-annotated images, e-commerce platforms can provide personalized recommendations, ultimately driving sales and improving customer satisfaction.
4. Agriculture
In agriculture, image annotation is used for monitoring crop health and detecting diseases. By training models on annotated images, farmers can receive timely insights to improve yield and reduce waste.
The Future of Image Annotation in Machine Learning
As machine learning technologies continue to evolve, the demand for high-quality image annotation will only accelerate. Here are some trends that are expected to shape the future of image annotation:
1. Increased Automation
The integration of AI into image annotation processes will streamline workflows, reduce time spent on manual labeling, and enhance accuracy. This evolution will empower businesses to tackle larger datasets more efficiently.
2. Enhanced Annotation Tools
Future developments in annotation tools will likely introduce novel features that simplify the annotation process, such as advanced machine learning-assisted labeling, which can predict and suggest annotations based on existing data.
3. Greater Emphasis on Quality
As industries become more reliant on machine learning, the emphasis on data quality will intensify. Companies like Keylabs.ai are already pioneering rigorous quality control measures to ensure that businesses receive the most reliable datasets for their development needs.
Conclusion
In conclusion, image annotation plays an indispensable role in the advancement of machine learning. From healthcare to automotive industries, the ability to accurately annotate images is key to the success of machine learning models. At Keylabs.ai, we are committed to providing state-of-the-art annotation tools and a robust platform to meet the ever-evolving needs of businesses. By investing in quality image annotation, organizations can unlock the full potential of their machine learning initiatives, driving forward innovations that can redefine entire industries.
Contact Us
If you are interested in revolutionizing your data annotation efforts, Keylabs.ai is here to assist you. Join us in spearheading the future of machine learning through high-quality image annotation.
image annotation machine learning